Machine Learning vs Computer Vision: How These Technologies Drive Innovation
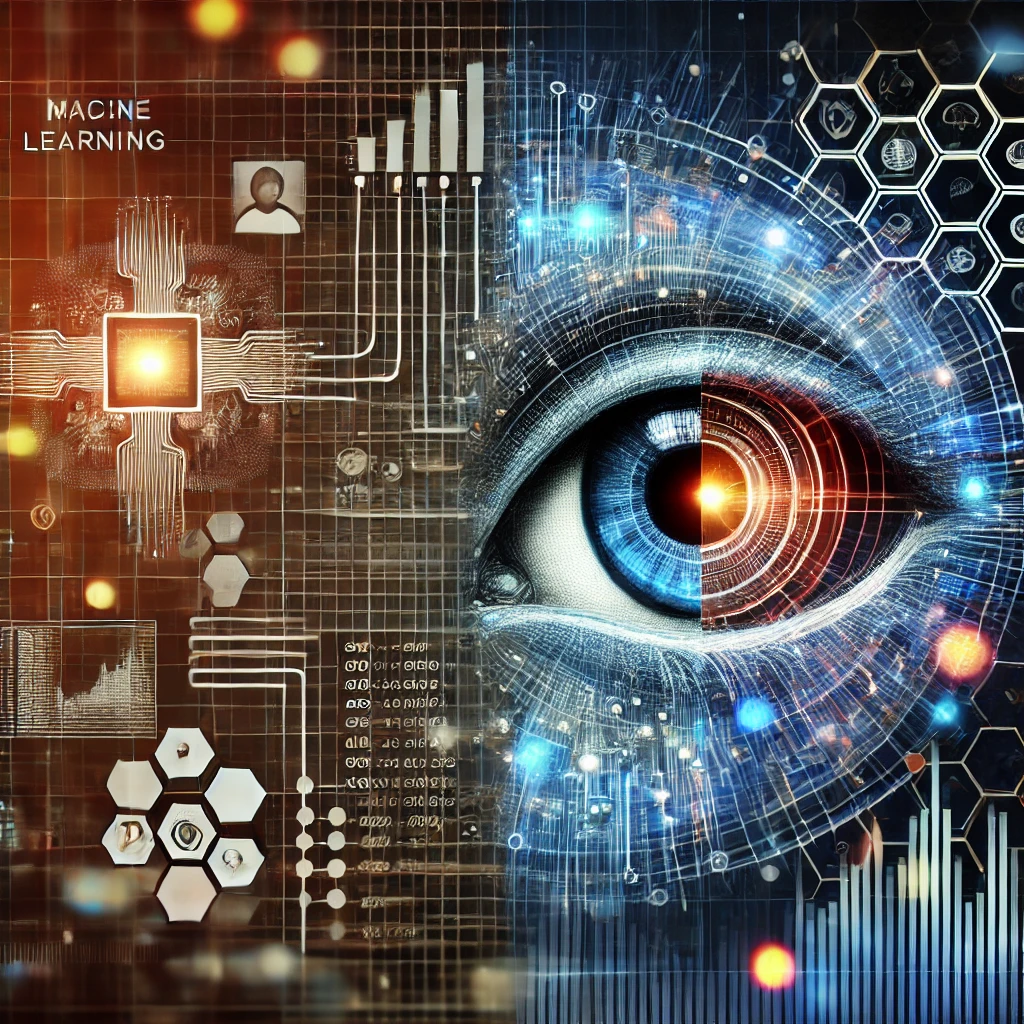
In the age of artificial intelligence (AI), machine learning (ML) and computer vision (CV) have emerged as powerful technologies revolutionizing industries across the globe. While these terms are often used interchangeably, they refer to distinct fields with unique applications. This article explores the differences between machine learning vs computer vision, their overlap, and the exciting possibilities each offers.
What is Machine Learning?
Machine learning is a subfield of AI that allows systems to learn from data and improve their performance over time. The core idea is to develop algorithms that can identify patterns, make decisions, and predict outcomes without being explicitly programmed. ML techniques, like supervised learning, unsupervised learning, and reinforcement learning, are widely used in fields like data science, natural language processing, and autonomous systems.
What is Computer Vision?
Computer vision, a subset of machine learning, enables machines to interpret and process visual data from the real world. CV systems analyze images and videos to extract meaningful information. With applications in image recognition, object detection, and facial recognition, computer vision is fundamental to technologies such as self-driving cars, medical imaging, and smart surveillance.
Machine Learning vs Computer Vision: Key Differences
Although closely related, machine learning and computer vision serve different purposes.
-
Data Type:
Machine learning can work with diverse types of data, including text, numbers, and images, while computer vision specifically deals with visual data. -
Purpose:
Machine learning focuses on improving decision-making through algorithms trained on data. In contrast, computer vision emphasizes enabling machines to “see” and interpret images or videos. -
Algorithms:
Computer vision often relies on machine learning algorithms, particularly deep learning and convolutional neural networks (CNNs), to process visual data.
How They Complement Each Other
While distinct, machine learning and computer vision often work together. For example, in a computer vision task like image classification, machine learning algorithms are used to train the model to recognize patterns in images. This synergy has led to advancements in fields such as healthcare, autonomous vehicles, and augmented reality (AR).
Applications of Machine Learning
Machine learning’s flexibility allows it to be applied across various sectors:
-
Healthcare:
Disease prediction models based on patient data. -
Finance:
Fraud detection algorithms for banks. -
Marketing:
Predictive analytics for customer behavior.
Applications of Computer Vision
Computer vision’s unique ability to interpret visual data opens up innovative applications, including:
-
Automotive:
Enabling autonomous vehicles to understand their surroundings. -
Security:
Facial recognition and surveillance systems. -
Retail:
Automated inventory management using image recognition.
Challenges and Future Directions
Both machine learning and computer vision face challenges related to data quality, privacy concerns, and computational costs. However, ongoing advancements in AI hardware and software continue to push the boundaries of what’s possible.
In the debate of machine learning vs computer vision, it’s clear that these technologies, while distinct, complement each other in powerful ways. Machine learning gives AI the ability to learn and adapt, while computer vision equips machines with the ability to see and interpret the world. Together, they form the backbone of transformative innovations that are shaping the future of industries globally.